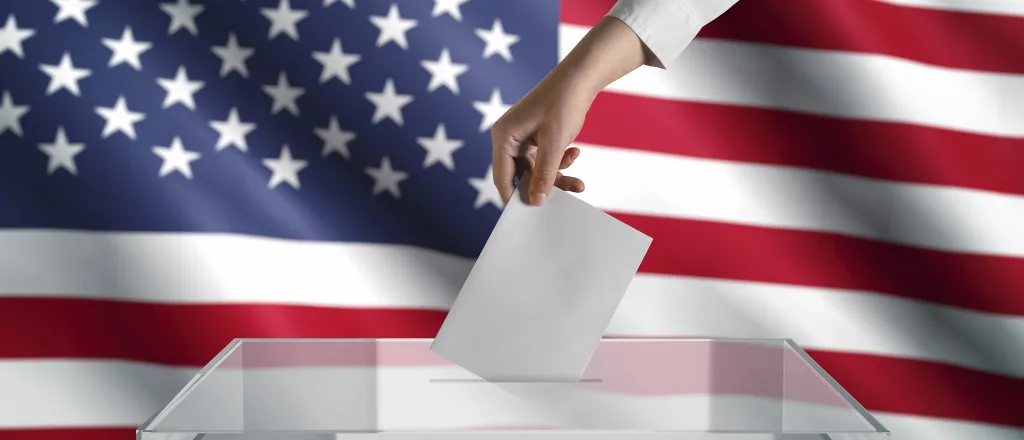
How the polls could have caught 'surprise' victories like Trump's
© iStock - sefa ouzel
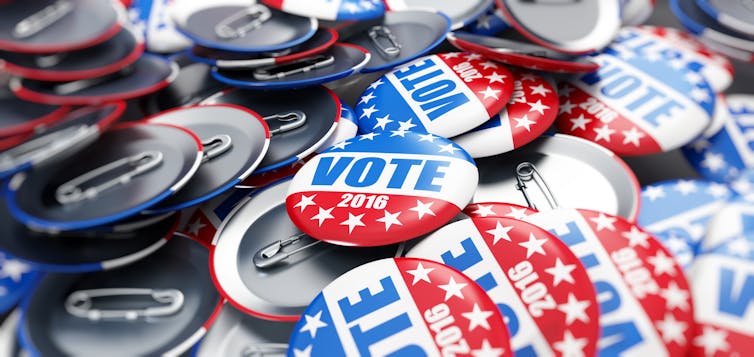
Fred Wright, North Carolina State University
The election of Donald Trump to the U.S. presidency surprised almost everyone, including apparently Trump himself.
On the morning after the 2016 election, my teenage son made snarky comments about the state of polling and statistical science. As a trained statistician, I took offense. However, I had no background in political science and really no idea what had gone “wrong.”
So I decided to put him to work, gathering and entering vote totals and poll data from 2016 and past elections, to judge for ourselves. In our analysis, we examined the performance of presidential poll-based predictions and proposed a new, improved model.
The 2016 election caused considerable hand-wringing over the state of opinion polling. However, the best evidence is that current polling is generally sound, but tune-ups to one particular aspect of how polls are collected – notably the practice of aggregating poll data – would be helpful.
Polling versus prediction
After the unexpected election outcome, most observers concluded that the polls, on average, underestimated support for Trump. Such a systematic error is known as “polling bias.” Numerous reports and think pieces have tried to explain the bias, pinpointing specific problems in how polls assessed likely voters and were weighted by voters’ education levels. Public misunderstanding of the concept of uncertainty also played a role.
To understand the issues, it’s important to recognize the distinction between polls, which represent samples of individuals at a particular time using a particular methodology, and poll aggregation.
Poll results can vary greatly, depending on who is sampled and what methods the pollsters use to weight respondents to account for nonrepresentative sampling, or assess who is likely to vote. With more and more people not answering pollsters and using cellphones, it’s an amazing testament to the field that opinion polling still works as well as it does.
When it comes to predicting an election, one must reconcile the often disparate poll results. That’s the role of poll aggregation sites, such as FiveThirtyEight, The Upshot and HuffPost, which average recent polls to produce a consensus.
For presidential elections, the sites go further and make predictions for each state, to tally a final electoral college outcome. Flashy graphics and accessible content make the sites hugely popular, driving public perception of the likely outcome.
Did the poll aggregators really miss?
Our own research suggests that the polling bias was actually not very large – that is, pollsters may have underestimated the support for Trump but not to a large degree. However, due to a statistical quirk, the prediction models were unable to recognize the dropping support for Hillary Clinton just prior to the election.
We examined the state-level predictions across all 50 states, plus D.C., in 2016, as well as their stated uncertainty.
We found that FiveThirtyEight and The Upshot showed statistical bias and overestimated support for Clinton, but with enough uncertainty that their probabilities left room for a Trump victory. HuffPost had similar state-level predictions, but was overconfident in these predictions, markedly overestimating the chance of a Clinton victory.
When the polling data up the eve of the election were fully taken into account, we estimated the chance of a Trump victory as at least 47 percent. The main novelty in our approach was to use polling data from multiple states to “fill in” the sparse information from state-level polling. In contrast, the popular poll aggregation sites gave much lower chances, ranging from 2 percent on HuffPost to about 29 percent on FiveThirtyEight.
An alternate explanation
But why would the polls have biased against Trump in the first place? An extensive report from the American Association for Public Opinion Research (AAPOR), an association for public opinion and survey research professionals, examined a number of possibilities, dismissing some popular theories.
Our own analysis suggests an additional possibility, hinted at in the AAPOR report: The polls weren’t highly biased and were roughly correct at the time. However, pollsters conducted too few state-level polls just prior to the election.
Remember, poll aggregators must average several polls to make a good prediction. Due to sparse state-level polling, predictions were “stuck” on values from about two weeks prior to the election, when support for Clinton had been higher.
Note that this sparse polling scenario indicates that polling methods are generally sound, although more frequent polling of swing states would be helpful.
Our rationale also explains why the estimate of the popular vote – 3.3 percent estimated margin for Clinton versus 2.1 percent actual – was largely accurate. National polls were conducted more frequently, and so the national averaging could include polls closer to the election.
Can pollsters do better?
The voter environment in 2016 election was unusual, with a sharp drop in support for the leading candidate prior to the election. Although it’s tempting to attribute the drop to then-FBI Director James Comey’s letter regarding an investigation into Clinton’s email server, we and others have noted that the drop in support for Clinton started in mid-October.
Although the 2016 experience was unusual, we proposed a statistical model designed to be sensitive to a national trend. The model combines information across numerous states, instead of relying only on polling within each state. The model estimates of the Democratic-Republican vote spread and overall win probabilities for the 90 days leading to an election.
Although our analysis was conducted after the election, we plan to try it out in 2020 in real time.
Fred Wright, Director of the Bioinformatics Research Center, North Carolina State University
This article is republished from The Conversation under a Creative Commons license. Read the original article.