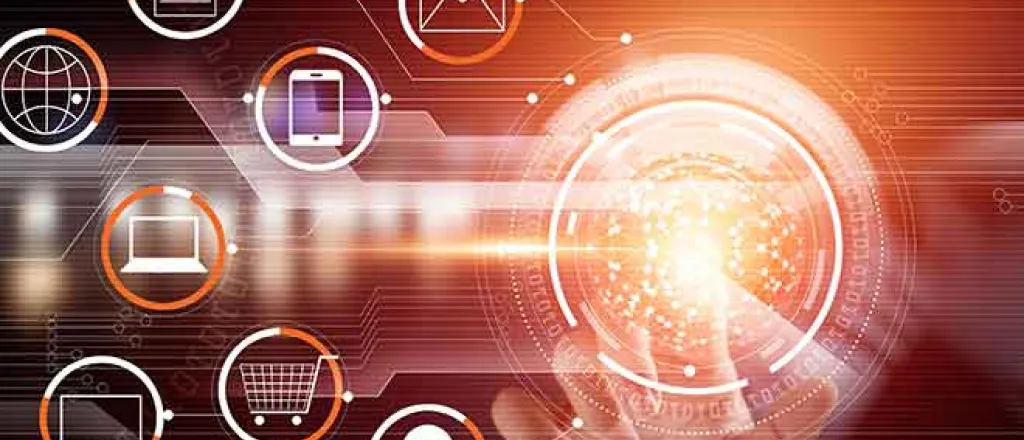
Most Americans don't realize what companies can predict from their data
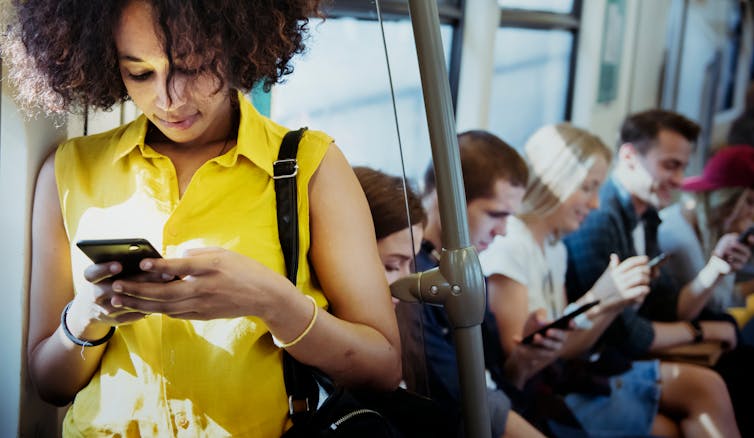
Emilee Rader, Michigan State University
Sixty-seven percent of smartphone users rely on Google Maps to help them get to where they are going quickly and efficiently.
A major of feature of Google Maps is its ability to predict how long different navigation routes will take. That’s possible because the mobile phone of each person using Google Maps sends data about its location and speed back to Google’s servers, where it is analyzed to generate new data about traffic conditions.
Information like this is useful for navigation. But the exact same data that is used to predict traffic patterns can also be used to predict other kinds of information – information people might not be comfortable with revealing.
For example, data about a mobile phone’s past location and movement patterns can be used to predict where a person lives, who their employer is, where they attend religious services and the age range of their children based on where they drop them off for school.
These predictions label who you are as a person and guess what you’re likely to do in the future. Research shows that people are largely unaware that these predictions are possible, and, if they do become aware of it, don’t like it. In my view, as someone who studies how predictive algorithms affect people’s privacy, that is a major problem for digital privacy in the U.S.
How is this all possible?
Every device that you use, every company you do business with, every online account you create or loyalty program you join, and even the government itself collects data about you.
The kinds of data they collect include things like your name, address, age, Social Security or driver’s license number, purchase transaction history, web browsing activity, voter registration information, whether you have children living with you or speak a foreign language, the photos you have posted to social media, the listing price of your home, whether you’ve recently had a life event like getting married, your credit score, what kind of car you drive, how much you spend on groceries, how much credit card debt you have and the location history from your mobile phone.
It doesn’t matter if these datasets were collected separately by different sources and don’t contain your name. It’s still easy to match them up according to other information about you that they contain.
For example, there are identifiers in public records databases, like your name and home address, that can be matched up with GPS location data from an app on your mobile phone. This allows a third party to link your home address with the location where you spend most of your evening and nighttime hours – presumably where you live. This means the app developer and its partners have access to your name, even if you didn’t directly give it to them.
In the U.S., the companies and platforms you interact with own the data they collect about you. This means they can legally sell this information to data brokers.
Data brokers are companies that are in the business of buying and selling datasets from a wide range of sources, including location data from many mobile phone carriers. Data brokers combine data to create detailed profiles of individual people, which they sell to other companies.
Combined datasets like this can be used to predict what you’ll want to buy in order to target ads. For example, a company that has purchased data about you can do things like connect your social media accounts and web browsing history with the route you take when you’re running errands and your purchase history at your local grocery store.
Employers use large datasets and predictive algorithms to make decisions about who to interview for jobs and predict who might quit. Police departments make lists of people who may be more likely to commit violent crimes. FICO, the same company that calculates credit scores, also calculates a “medication adherence score” that predicts who will stop taking their prescription medications.
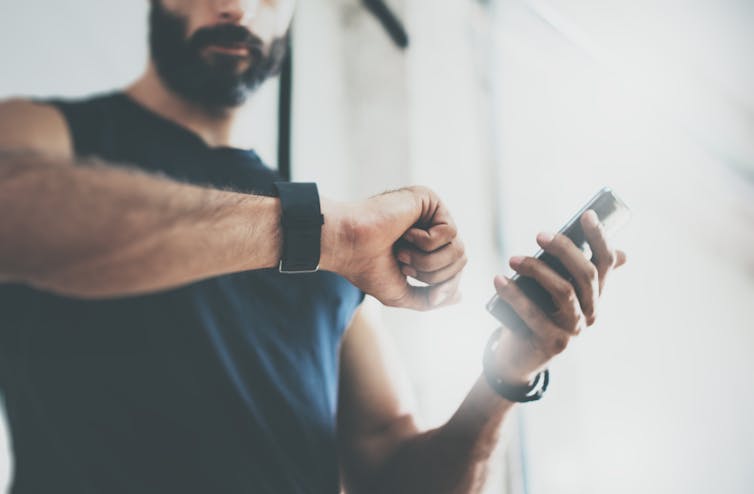
How aware are people about this?
Even though people may be aware that their mobile phones have GPS and that their name and address are in a public records database somewhere, it’s far less likely that they realize how their data can be combined to make new predictions. That’s because privacy policies typically only include vague language about how data that’s collected will be used.
In a January survey, the Pew Internet and American Life project asked adult Facebook users in the U.S. about the predictions that Facebook makes about their personal traits, based on data collected by the platform and its partners. For example, Facebook assigns a “multicultural affinity” category to some users, guessing how similar they are to people from different race or ethnic backgrounds. This information is used to target ads.
The survey found that 74 percent of people did not know about these predictions. About half said they are not comfortable with Facebook predicting information like this.
In my research, I’ve found that people are only aware of predictions that are shown to them in an app’s user interface, and that makes sense given the reason they decided to use the app. For example, a 2017 study of fitness tracker users showed that people are aware that their tracker device collects their GPS location when they are exercising. But this doesn’t translate into awareness that the activity tracker company can predict where they live.
In another study, I found that Google Search users know that Google collects data about their search history, and Facebook users are aware that Facebook knows who their friends are. But people don’t know that their Facebook “likes” can be used to accurately predict their political party affiliation or sexual orientation.
What can be done about this?
Today’s internet largely relies on people managing their own digital privacy.
Companies ask people up front to consent to systems that collect data and make predictions about them. This approach would work well for managing privacy, if people refused to use services that have privacy policies they don’t like, and if companies wouldn’t violate their own privacy policies.
But research shows that nobody reads or understands those privacy policies. And, even when companies face consequences for breaking their privacy promises, it doesn’t stop them from doing it again.
Requiring users to consent without understanding how their data will be used also allows companies to shift the blame onto the user. If a user starts to feel like their data is being used in a way that they’re not actually comfortable with, they don’t have room to complain, because they consented, right?
In my view, there is no realistic way for users to be aware of the kinds of predictions that are possible. People naturally expect companies to use their data only in ways that are related to the reasons they had for interacting with the company or app in the first place. But companies usually aren’t legally required to restrict the ways they use people’s data to only things that users would expect.
One exception is Germany, where the Federal Cartel Office ruled on Feb. 7 that Facebook must specifically ask its users for permission to combine data collected about them on Facebook with data collected from third parties. The ruling also states that if people do not give their permission for this, they should still be able to use Facebook.
I believe that the U.S. needs stronger privacy-related regulation, so that companies will be more transparent and accountable to users about not just the data they collect, but also the kinds of predictions they’re generating by combining data from multiple sources.
Emilee Rader, Associate Professor of Media and Information, Michigan State University
This article is republished from The Conversation under a Creative Commons license. Read the original article.